Understanding Image Labeling: A Key Element in Data Annotation
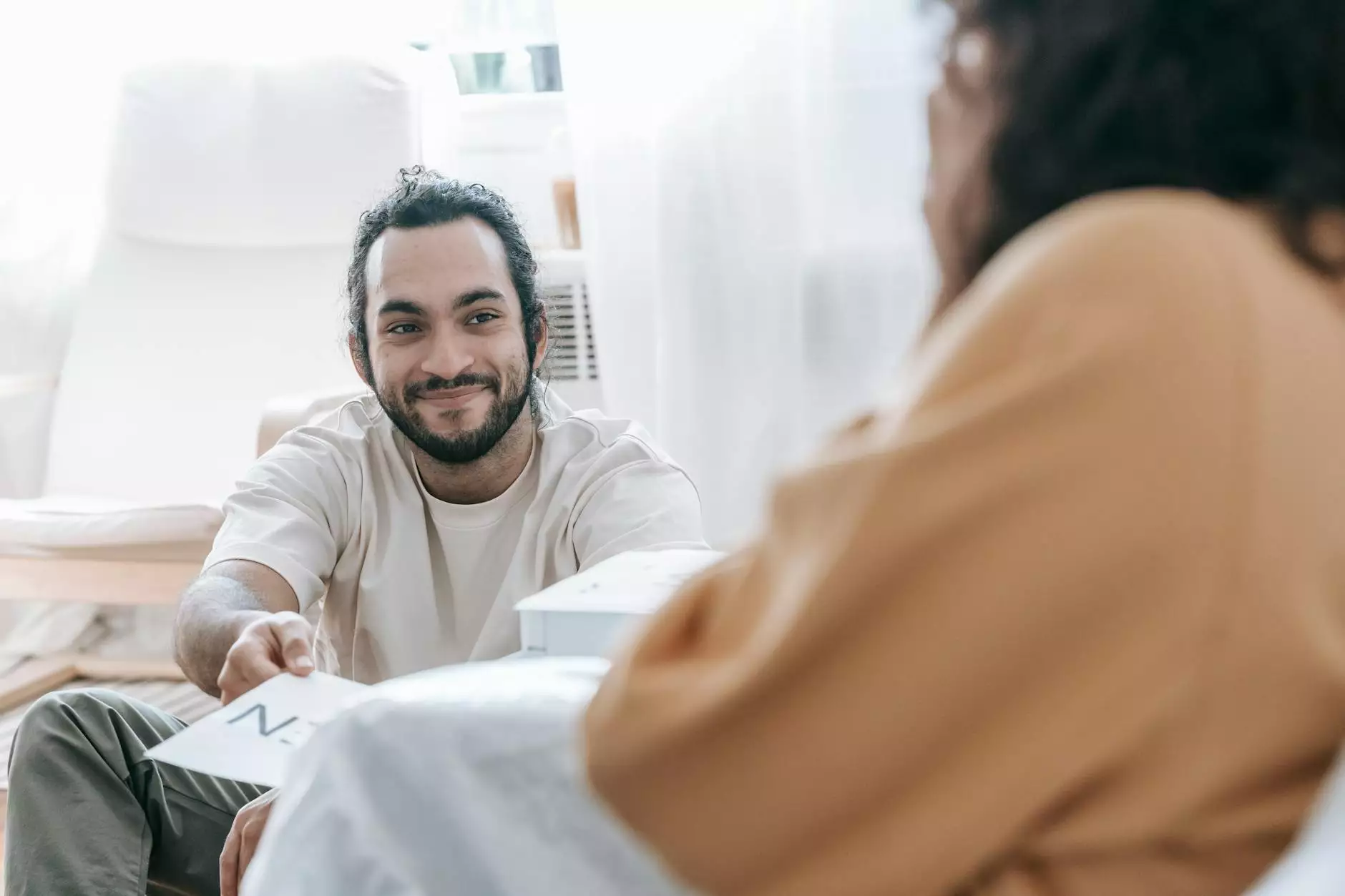
Image labeling is a crucial process in the field of machine learning and artificial intelligence. It refers to the practice of identifying and categorizing objects within digital images. This process is fundamental to training algorithms to recognize patterns, making it indispensable for various applications such as autonomous driving, facial recognition, and inventory management.
Why Image Labeling Matters
With the exponential growth of data in the digital world, the importance of accurately labeling images has never been greater. Proper image labeling enables businesses and researchers to:
- Improve Model Accuracy: Well-labeled images provide reliable datasets that enhance the predictive performance of machine learning models.
- Enhance Data Usability: Labeling data correctly ensures that it can be utilized effectively for various analytical purposes.
- Facilitate Compliance: Many industries are required to follow strict data governance guidelines which necessitate accurate data handling practices.
How Image Labeling Works
The process of image labeling involves several key steps:
1. Data Collection
The first step involves gathering the images that you want to label. This can include any variety of images that are relevant to your business or research project.
2. Choosing the Right Tool
Selecting an appropriate data annotation tool is crucial. Tools like Keylabs.ai offer sophisticated functionalities that simplify the labeling process through user-friendly interfaces and features like:
- Automated labeling suggestions
- Collaborative workflows
- Integration capabilities with existing data systems
3. Labeling Techniques
The actual labeling process can utilize a variety of techniques, dependent on the requirements of the project:
- Bounding Boxes: Drawing rectangles around objects of interest in an image.
- Segmentation: Dividing an image into multiple segments for more precise labeling.
- Point Annotation: Marking specific points to signify object presence.
4. Quality Assurance
After the labeling process, it’s essential to conduct quality checks. This ensures that errors are minimized and the data remains trustworthy for model training.
The Role of Keylabs.ai in Advanced Image Labeling
Keylabs.ai provides a state-of-the-art data annotation platform that alleviates the challenges involved in image labeling. Here’s why our platform stands out:
Comprehensive Toolset for Efficient Labeling
Our platform encompasses a variety of tools designed to maximize productivity and accuracy, including:
- Intelligent Automation: Automated labeling processes that reduce manual effort, allowing for quick turnaround times.
- Versatile Annotation Options: Flexible annotation configurations to meet the needs of different image types and projects.
- User-Friendly Interface: Designed for both technical and non-technical users, making it accessible for all team members.
Scalability and Flexibility
As your business grows, so does the need for data annotation. Keylabs.ai offers scalable solutions that evolve with your requirements, allowing you to manage increased data loads without compromising quality.
Industry Applications of Image Labeling
The implications of effective image labeling reach various industries, enhancing efficiency and accuracy across the board:
1. Automotive
In the automotive industry, image labeling plays a vital role in developing autonomous vehicles. Labels help machines learn to recognize pedestrians, traffic signs, and road conditions, which is essential for ensuring safety.
2. Healthcare
For medical imaging, precise image labeling can support diagnostic processes. By accurately identifying anomalies in scans, AI systems can assist in early disease detection and enhance patient outcomes.
3. Retail
Retailers use image labeling for inventory management and e-commerce platforms. Annotations can help in classifying products and recognizing items to streamline operations and improve customer experience.